High Deductible Healthcare Plans (HDHPs) have become the preferred insurance option for many Americans primarily due to their lower premiums. They are also a popular health insurance plan offered by private-sector employers. In 2022, more than half of U.S. private-sector workers (53.6%) were enrolled in HDHPs.
However, HDHPs have a higher deductible than traditional insurance plans, meaning individuals must cover more healthcare expenses out of pocket before the insurance company starts contributing.
In this blog post, we will discuss the rising popularity of HDHPs and their implications for RCM providers and Extended Business Offices (EBOs). Additionally, we will explain why Conversational AI technology is a game-changer for early-out collections.
Why Are High Deductible Healthcare Plans (HDHPs) Becoming Popular?

Rising Health Insurance Costs
With rising health insurance costs and hospital charges, people are opting for HDHPs, which have lower premiums. The American Medical Association (AMA) reports that healthcare costs are climbing at approximately 4.5% annually. In 2019, healthcare spending in the United States increased by 4.6%, reaching a staggering $3.8 trillion nationwide, equating to an average of $11,582 per person. This increase aligns closely with the rates seen in 2018 (4.7%) and slightly surpasses those of 2017 (4.3%).
Besides the ongoing trend of healthcare costs inching upward, short-term factors have also played a significant role. Many U.S. residents experienced faster-than-average increases in their health insurance costs in 2021, as insurance companies and healthcare providers raised costs post-pandemic.
As a result, over half of all U.S. workers were enrolled in high-deductible health plans (55.7%). Enrollment for HDHPs has risen for the eighth consecutive year in 2023, the highest enrollment rate since 2012.
HDHPs are Cheaper for Employers
Employers regularly seek strategies to offer stable benefits while reducing costs. This practice enhances their competitiveness in the job market while keeping expenses in check.
According to a recent Mercer study, larger employers spend an average of $84 per month on High Deductible Healthcare Plans (HDHPs) per employee, compared to $132 per month for traditional Preferred Provider Organization (PPO) plans. This shift towards HDHPs translates to a significant 37% reduction in costs per employee, with greater savings realized by larger companies.
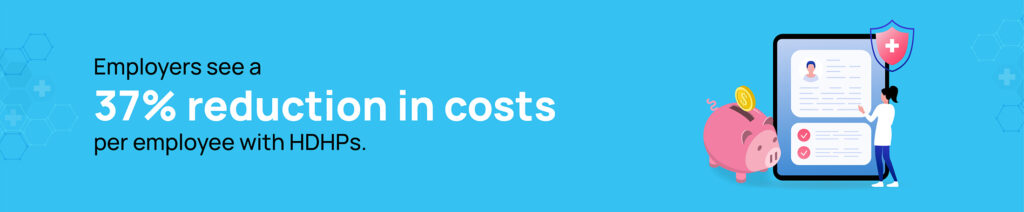
Flexible Coverages
Beyond cost savings, HDHPs offer enhanced flexibility in healthcare coverage. Unlike Health Maintenance Organizations (HMOs), HDHPs typically impose fewer restrictions, granting individuals greater freedom to select their preferred service providers. This increased flexibility removes hurdles from the healthcare decision-making process, empowering individuals to make more informed choices about their healthcare options.
HDHPs = Savings
HDHPs can also provide additional savings opportunities for individuals. HDHP is the sole Health Savings Account (HSA)-eligible health plan that helps with additional savings. With an HDHP, individuals can establish an HSA to benefit from tax-free saving, investing, and spending on healthcare expenses. HSAs offer several advantages, including the ability to carry over funds yearly without expiration. Unlike other types of accounts, HSAs are owned by the individual and can be transferred between jobs and healthcare providers. Furthermore, HSAs serve as an additional retirement account.
This ownership of HSA funds provides stability amidst the ever-changing healthcare landscape, allowing individuals to retain their accounts even as they transition to different health plans each year.
What Does This Mean For RCM Providers and EBOs?
The increase in demand and adoption of HDHPs has significant implications for Revenue Cycle Management (RCM) providers and External Business Offices (EBOs) especially when collecting self-pay dues in early-out collections.
Let’s explore how this trend affects early-out collections and alters the revenue cycle for these businesses.
Increased Patient Self-Pay Dues = Delayed Cash Flow
HDHPs typically come with higher deductibles, meaning patients are responsible for a larger portion of their healthcare expenses upfront before insurance coverage kicks in. As a result, patients may delay or struggle to pay their medical bills, leading to a higher volume of outstanding balances in early-out collections.
With this delay, the revenue cycle for RCM providers and EBOs may lengthen as they wait longer to receive patient payments. This impact on the cash inflow can strain liquidity and hinder financial planning efforts.
Challenges in Collecting Payments
RCM providers and EBOs may encounter challenges collecting payments from patients with HDHPs. The increased self-pay responsibilities require increased engagement efforts from RCMs/EBOs to reach patients and collect payments. There is also a higher denial rate for self-pay dues. This requires additional resources, such as spending time resolving billing disputes and answering queries.
Need to Enhance Patient Communication
RCM providers and EBOs must prioritize communication to ensure patients are aware of their self-pay dues. This may involve explaining insurance coverage, clarifying billing statements, and offering payment plan options to facilitate timely collections.
Focus on Proactive Payment Strategies
RCM providers and EBOs must adopt proactive payment strategies to streamline billing and payment processes in response to the challenges posed by HDHPs. This approach facilitates the retrieval of self-pay obligations and fosters trust among patients.
How Does Conversational AI Help Expedite Early-Out Collections?
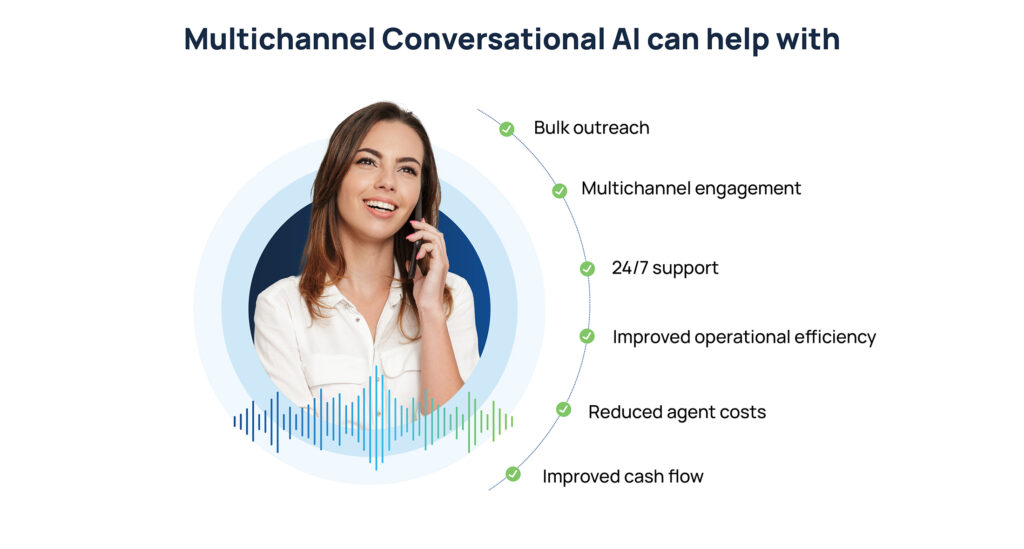
Skit.ai’s Multichannel Conversational AI solution can aid RCMs and EBOs by expediting early-out collections. Here’s how:
Bulk Outreach and Multichannel Engagement
Skit.ai’s AI bot can initiate outreach to patients and engage with them in the following days via multiple channels, such as phone calls (Voice AI), text messages, emails, and chatbots, ensuring effective communication and engagement from the outset. It can manage complex, multi-turn conversations with patients across all channels, maintaining context seamlessly. The Voice AI solution engages with patients in human-like conversations. This ensures meaningful engagement with them and, at the same time, offers scalability to RCM providers to reach out to numerous patients in bulk, thus helping mitigate potential payment delays and improving patient satisfaction.
More Than Just a Call; Available at Patient’s Beck and Call
Skit.ai’s AI bot can authenticate patients, clarify bill breakdowns, answer patient queries, facilitate on-call payments and text-based payment links, and even set up payment plans, enhancing convenience and reducing barriers to receiving payment.
Enhanced Cash Flow
With more outreach, faster query resolution, and seamless payment options (on-call payments and text-based payment links), Skit.ai enables RCM and EBOs to do more early-out collections of self-pay dues.
Improved Efficiency and Reduced Agent Costs
Skit.ai’s AI bot augments human efforts by automating repetitive and time-consuming tasks, enabling RCM staff to focus on resolving complex disputes and providing personalized patient assistance.
Through automation, operational expenses are reduced, revenues are maximized, and overall productivity within the organization is enhanced. Additionally, this results in decreased staffing needs and reduced training costs for RCM providers and EBOs.
Conclusion
The surge in high-deductible healthcare plans (HDHPs) underscores a shift in early-out collections. The rise in patient self-pay dues under HDHPs requires increased engagement efforts and proactive payment strategies to streamline billing processes and enhance revenue cycles. Additionally, adopting Conversational AI solutions offers a promising avenue for overcoming these challenges.
Conversational AI improves efficiency, enhances cash flow, and reduces costs for RCM providers and EBOs by facilitating bulk outreach, providing comprehensive patient assistance, and automating repetitive tasks.
Curious to learn more about how Conversational AI can help you gain a competitive edge over your competitors? Book a free demo with one of our experts.